Detecting AI-Generated Text: A Look at the Challenges and Mitigations
|
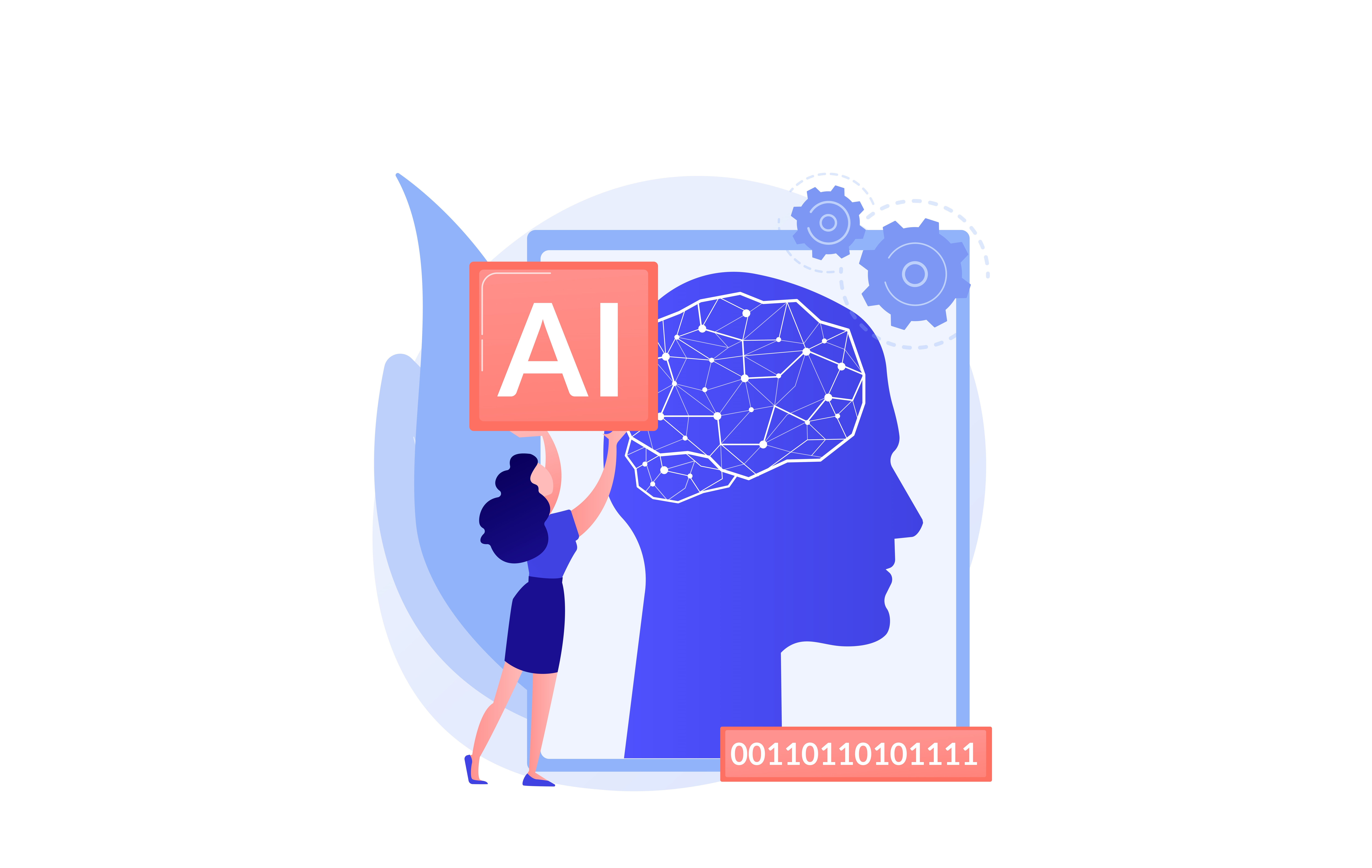
The emergence of artificial intelligence (AI) has made a significant impact on how people communicate, work, and live their lives. From natural language processing to facial recognition, AI technology is being used in more industries than ever before.
But with this increased usage comes an increased need for reliable detection of AI-generated text. This article will take a look at the methods and their challenges for detecting artificial intelligence-generated text.
AI technology has advanced rapidly over the last few years, allowing it to generate increasingly convincing texts that can pass off as human written work. These AI-generated texts are becoming more prevalent in areas such as news articles, blog posts, and even books. As a result, detecting these texts is becoming increasingly important for organizations looking to ensure accuracy and trustworthiness in their written communications.
While it is impossible to reliably detect all AI-written text using automated methods alone, several techniques can be used to help better identify when an article or other piece of writing was generated by an artificial intelligence system rather than a human author.
Strategies For Detecting AI-Generated Text
There are a few strategies that are being employed to detect AI-generated text.
Statistical Analysis
One technique commonly used for detecting AI-generated text involves analyzing statistical properties such as word choice frequencies and sentence length distributions within a given document or set of documents for clues about its authorship status (i.e., machine vs. human). For example, if a document contains words or phrases that appear more often than would normally be expected from natural language communication, this could indicate it was produced by an artificial source rather than by humans (as these systems often rely on preprogrammed word banks). Furthermore, if one examines sentence lengths within these documents, one may notice that they consistently fall into specific patterns, which could serve as evidence of their machine origin status, as machines usually follow set rules when producing content, whereas humans do not have such constraints on their creativity levels when writing freely flowing prose pieces. Similarly, grammar-checking tools can help flag up sections where syntax errors arise frequently - another indicator that something might have been generated by an algorithm rather than people typing out sentences manually from scratch.
Stylometry Analysis
Another way of attempting to distinguish between machine versus human authorship is through stylometry - analyzing certain aspects related specifically to written style such as sentence structures/phrases used, etc., which can then provide clues about who wrote what based upon how similar those patterns match up against known works created either by machines or humans and analyzed beforehand (e.g., using database search algorithms). Furthermore, there are now some deep learning models being developed that use neural networks trained on large datasets containing both machine/human authored pieces so that they can learn how each type differs from one another enough so that it knows whether something should be classified accordingly once tested against new samples provided afterward as well - though these models still require refinement before they become robust enough for widespread use currently!.
Contextual Analysis
One way organizations can detect whether a piece of content was written by an AI algorithm is through contextual analysis which looks at how words are used within sentences to assess their meaning as well as other factors such as capitalization trends and punctuation usage patterns which may indicate if something was written mechanically versus manually written by someone familiar with grammar rules. Additionally, the contextual analysis also considers other elements such as tone shifts between sentences, which may suggest different authors wrote different parts due to poor stitching together when multiple sources were combined via machine learning algorithms like GPT3 (Generative Pre-trained Transformer 3).
Introducing Kafkai
Kafkai, our SaaS that generates unique content, especially for SEO leverages different types of generative AI frameworks using different models that we use specific to a particular niche. If you require quick and unique content for your blog or online marketing strategy, try out Kafkai. We also have a generous affiliate program of up to 50% for you to share with your customers.
Contact us here for a trial account and free consultation.
Natural Language Processing (NLP) tools
Natural language processing tools such as sentiment analysis allow organizations not only to analyze how people feel about certain topics but also determine if certain pieces were generated using an NLG model rather than manually composed by a human author because they will pick up on common phrases used regularly in machine learning generated texts that would otherwise not be present had it been written organically. These tools will also often highlight areas where there might be discrepancies between two pieces that look identical but have subtle differences that indicate one was artificially created while the other wasn't.
There are also online tools that you can try to use, such as writer.com's AI Content Detector or an implementation of a paper by Stanford researchers called DetectGPT. DetectGPT is based on the assumption that A- written text follows a specific pattern that is inherent in the language model that generated it, but our limited, random tests yielded inconclusive results, with frequent false negatives and positives. As we wrote above, it is still impossible to reliably detect if an article was written by AI.
Throw warm bodies at the problem
When all else fails, having humans review each piece individually remains one surefire way to guarantee accuracy when trying to detect whether something has been generated using an artificial intelligence algorithm. By having experts review each piece manually, they can spot signs that indicate potential machine learning generation, like unnatural word choices, overly complex structures, repetition, etc. Additionally, this method allows for more subjective interpretations, allowing experts to make decisions based on their experience rather than relying solely on automated methods. At the same time, we also need to note that studies have found that humans did not do any significantly better at detecting AI written content compared to automated methods.
In Summary
Although no single approach will perfectly detect all cases where content is artificially created. If one wishes to try and detect particular content written by AI, multiple approaches need to be combined. However, we believe that this is an incorrect approach to addressing this so-called issue. The real question we should be asking instead is this: Why not AI-generated content? AI-generated contents have its place in this world, and instead of not leveraging them to make our work and lives more efficient and better, we should use them where it can shine the brightest and use other strategies for instances where they don't. In case you know that you definitely need human-written content, we also have Karya Mart, a market for human-written SEO Optimized Articles, Ready To Go.